基于阿里通義 Qwen3 混合推理模型才是優(yōu)化 RAG 和 MCP 的最佳范式 原創(chuàng)
阿里巴巴推出了全新的 Qwen3 系列模型。令人驚嘆的是,在短短的12個(gè)小時(shí)內(nèi),這個(gè)系列在 GitHub 上的星標(biāo)數(shù)就超過了17,000個(gè),而在 Hugging Face 上的下載量更是達(dá)到了每小時(shí)23,000次的高峰。
更讓人興奮的是,Qwen3 系列這次一共推出了八種不同的模型,包括兩款 MoE 模型:Qwen3-235B-A22B(2350多億總參數(shù)、 220多億激活參),以及 Qwen3-30B-A3B(300億總參數(shù)、30億激活參數(shù));以及六個(gè) Dense 模型:Qwen3-32B、Qwen3-14B、Qwen3-8B、Qwen3-4B、Qwen3-1.7B和Qwen3-0.6B。
它們都是混合推理模型,這意味著它們既能快速思考,也能深入思考。這些模型在推理能力、遵循指令、調(diào)用工具以及多語言處理等方面都有了顯著的提升,而且它們還刷新了所有國產(chǎn)模型以及全球開源模型的性能紀(jì)錄。
接下來結(jié)合 Qwen3 構(gòu)建 RAG 和 MCP 詳細(xì)分析之。
1、Qwen3 更強(qiáng),更多選擇,更低門檻,更適合企業(yè)落地
Qwen3 系列模型亮點(diǎn):混合推理、云端與本地、小尺寸高能、多語言支持
(1)混合推理模型:Qwen3 系列的所有模型都是推理與非推理相結(jié)合的混合模型,滿足老板對(duì)成本和性能的雙重要求。
(2)MoE 與 Dense 模型:系列包含兩款 MoE(專家混合)模型和六款Dense(稠密)模型,前者適合云端部署,后者在本地表現(xiàn)更優(yōu)。
(3)小尺寸高能:基于小尺寸的能力升級(jí),只需4張 H20 顯卡即可部署完整的 Qwen3 模型。
(4)MCP 與多語言:支持 MCP 和多語言能力,降低開發(fā)成本。
詳解 Qwen3 系列模型的四大關(guān)鍵詞:
第一、混合推理模型:Qwen3 系列全部采用混合推理模型,兼具推理(深思熟慮)與非推理(快速反應(yīng))能力,符合行業(yè)發(fā)展趨勢,平衡算力成本與輸出效果。
第二、MoE 與 Dense 模型:包括兩款 MoE 模型和六款 Dense 模型,MoE 模型適合云端,Dense 模型本地表現(xiàn)更佳。
第三、小尺寸高能:Qwen3 系列訓(xùn)練數(shù)據(jù)量達(dá)36T tokens,最大模型 Qwen3-235B-A22B 擁有2350多億總參數(shù),但部署成本不高,支持動(dòng)態(tài)量化,僅需4張H20顯卡。
第四、MCP 與多語言:Qwen3 系列支持 MCP,便于與外部數(shù)據(jù)庫、工具交互,同時(shí)支持119種語言和方言,服務(wù)全球開發(fā)者,助力企業(yè)全球業(yè)務(wù)拓展。
總結(jié):Qwen3 系列模型以其混合推理、云端與本地部署、小尺寸高能、多語言支持等特點(diǎn),非常適合企業(yè)場景中落地,幫助開發(fā)者構(gòu)建性能與成本全面可控的產(chǎn)品。
2、基于推理模式和非推理模式實(shí)現(xiàn) RAG 對(duì)比
RAG 的構(gòu)建流程和步驟詳細(xì)看這里《???別搞 GraphRAG 了,擁抱新一代 RAG 范式 DeepSearcher???》,這里不再贅述。下面通過一個(gè)具體問題詳細(xì)對(duì)比剖析。
基礎(chǔ)數(shù)學(xué)問題:甲和乙從同一地點(diǎn)出發(fā),甲先走2小時(shí),速度5km/h,乙以15km/h追趕,多久追上?
第一、基于 Qwen3 推理模式的參考代碼如下:
import os
import time
from openai import OpenAI
os.environ["DASHSCOPE_API_KEY"] = "sk-*************************"
client = OpenAI(
api_key=os.getenv("DASHSCOPE_API_KEY"),
base_url="https://dashscope.aliyuncs.com/compatible-mode/v1",
)
############################################
# Think
# 記錄開始時(shí)間
start_time = time.time()
stream = client.chat.completions.create(
# 模型列表:https://help.aliyun.com/zh/model-studio/getting-started/models
model="qwen3-235b-a22b",
messages=[
{"role": "system", "content": "You are a helpful assistant."},
{"role": "user", "content": "甲和乙從同一地點(diǎn)出發(fā),甲先走2小時(shí),速度5km/h,乙以15km/h追趕,多久追上?"},
],
# Qwen3模型通過enable_thinking參數(shù)控制思考過程(開源版默認(rèn)True,商業(yè)版默認(rèn)False)
extra_body={"enable_thinking": True},
stream=True,
)
answer_content = ""
for chunk in stream:
delta = chunk.choices[0].delta
if delta.content is not None:
answer_content += delta.content
print(answer_content)
# 記錄結(jié)束時(shí)間并計(jì)算總耗時(shí)
end_time = time.time()
print(f"\n\n總耗時(shí):{end_time - start_time:.2f}秒")
從答案的質(zhì)量來看,推理模式成功識(shí)別出了這是一個(gè)追及問題。它分析了題目中給出的條件,并提出了兩種不同的解題方法和正確的答案。這表明模型對(duì)這個(gè)問題進(jìn)行了深入的思考。特別是,它最后提供的 Markdown 格式答案,其中的數(shù)學(xué)公式顯示得非常精準(zhǔn)。
整個(gè)過程的代碼運(yùn)行時(shí)間是 35.73秒。
(下面是我們將模型生成的 Markdown 答案轉(zhuǎn)換成可視化圖像的截圖,為了讓讀者更容易理解)
第二、基于 Qwen3 非推理模式的參考代碼如下:
在以上代碼設(shè)置中,只需將 `"enable_thinking"` 設(shè)置為 `False`。
接下來,讓我們看看非推理模式是如何處理這個(gè)問題的:
非推理模式采用逐步求解的方法,使用了常規(guī)的相對(duì)速度法,并迅速得出了正確答案。
它的總耗時(shí)大約是:6.89秒,大約是推理模式耗時(shí)的五分之一??梢钥闯?,推理模式相比非推理模式會(huì)進(jìn)行更多的思考。
這種額外的思考可以使回答內(nèi)容更加豐富,邏輯性更強(qiáng)。但是,非推理模式的回答速度更快。這兩種模式在處理不同類型的問題時(shí)各有優(yōu)勢。因此,用戶可以根據(jù)自己的需求來選擇使用哪種模式。
3、MCP 的支持
第一、MCP 的支持
Qwen3 在工具調(diào)用能力方面表現(xiàn)出色。我們推薦使用 Qwen-Agent 來充分發(fā)揮 Qwen3 的 Agent 能力。Qwen-Agent 內(nèi)部封裝了工具調(diào)用模板和工具調(diào)用解析器,大大降低了代碼復(fù)雜性。
要定義可用的工具,您可以使用 MCP 配置文件,使用 Qwen-Agent 內(nèi)置的工具,或者自行集成其他工具。
MCP 功能支持的代碼如下所示:
from qwen_agent.agents import Assistant
# Define LLM
llm_cfg = {
'model': 'Qwen3-30B-A3B',
# Use the endpoint provided by Alibaba Model Studio:
# 'model_type': 'qwen_dashscope',
# 'api_key': os.getenv('DASHSCOPE_API_KEY'),
# Use a custom endpoint compatible with OpenAI API:
'model_server': 'http://localhost:8000/v1', # api_base
'api_key': 'EMPTY',
# Other parameters:
# 'generate_cfg': {
# # Add: When the response content is `<think>this is the thought</think>this is the answer;
# # Do not add: When the response has been separated by reasoning_content and content.
# 'thought_in_content': True,
# },
}
# Define Tools
tools = [
{'mcpServers': { # You can specify the MCP configuration file
'time': {
'command': 'uvx',
'args': ['mcp-server-time', '--local-timeznotallow=Asia/Shanghai']
},
"fetch": {
"command": "uvx",
"args": ["mcp-server-fetch"]
}
}
},
'code_interpreter', # Built-in tools
]
# Define Agent
bot = Assistant(llm=llm_cfg, function_list=tools)
# Streaming generation
messages = [{'role': 'user', 'content': 'https://qwenlm.github.io/blog/ Introduce the latest developments of Qwen'}]
for responses in bot.run(messages=messages):
pass
print(responses)
第二、軟切換機(jī)制
Qwen3 提供了一種軟切換機(jī)制,允許用戶在 enable_thinking=True 時(shí)動(dòng)態(tài)控制模型的行為。具體來說,可以在用戶提示或系統(tǒng)消息中添加 /think 和 /no_think 來逐輪切換模型的思考模式。在多輪對(duì)話中,模型會(huì)遵循最近的指令。
以下是一個(gè)多輪對(duì)話的示例:
from transformers import AutoModelForCausalLM, AutoTokenizer
class QwenChatbot:
def __init__(self, model_name="Qwen3-30B-A3B/Qwen3-30B-A3B"):
self.tokenizer = AutoTokenizer.from_pretrained(model_name)
self.model = AutoModelForCausalLM.from_pretrained(model_name)
self.history = []
def generate_response(self, user_input):
messages = self.history + [{"role": "user", "content": user_input}]
text = self.tokenizer.apply_chat_template(
messages,
tokenize=False,
add_generation_prompt=True
)
inputs = self.tokenizer(text, return_tensors="pt")
response_ids = self.model.generate(**inputs, max_new_tokens=32768)[0][len(inputs.input_ids[0]):].tolist()
response = self.tokenizer.decode(response_ids, skip_special_tokens=True)
# Update history
self.history.append({"role": "user", "content": user_input})
self.history.append({"role": "assistant", "content": response})
return response
# Example Usage
if __name__ == "__main__":
chatbot = QwenChatbot()
# First input (without /think or /no_think tags, thinking mode is enabled by default)
user_input_1 = "How many r's in strawberries?"
print(f"User: {user_input_1}")
response_1 = chatbot.generate_response(user_input_1)
print(f"Bot: {response_1}")
print("----------------------")
# Second input with /no_think
user_input_2 = "Then, how many r's in blueberries? /no_think"
print(f"User: {user_input_2}")
response_2 = chatbot.generate_response(user_input_2)
print(f"Bot: {response_2}")
print("----------------------")
# Third input with /think
user_input_3 = "Really? /think"
print(f"User: {user_input_3}")
response_3 = chatbot.generate_response(user_input_3)
print(f"Bot: {response_3}")
4、總結(jié)
總的來說,這次發(fā)布的 Qwen3 系列模型不僅在各個(gè)專業(yè)領(lǐng)域達(dá)到了最佳性能(SOTA),還特別注重實(shí)際應(yīng)用和工程化部署。它們?yōu)闃?gòu)建 RAG(檢索增強(qiáng)生成)或智能體(AI Agent)提供了很好的范例,幫助在成本和性能之間找到平衡點(diǎn)。
比如,與 DeepSeek 相比,Qwen3 模型的參數(shù)更少,這有助于降低部署成本;支持更多語言,增加了全球開發(fā)者的使用便利性;支持 MCP(模型上下文協(xié)議),增強(qiáng)了與其他系統(tǒng)的集成能力;還有混合推理模型的設(shè)計(jì),讓用戶可以自由選擇是否進(jìn)行深度推理,從而精確控制輸出成本。
在最近開源的 DeepSearcher 項(xiàng)目(由 Zilliz 開發(fā)的深度檢索和報(bào)告生成工具)中,現(xiàn)在 DeepSearcher 已經(jīng)迅速支持了 Qwen3 模型,幫助用戶找到性能和成本之間的最佳平衡點(diǎn)。
Github 地址:
??https://github.com/zilliztech/deep-searcher?tab=readme-ov-file??#configuration-details
本文轉(zhuǎn)載自??玄姐聊AGI?? 作者:玄姐
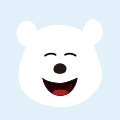