從零實現(xiàn)大模型-GPT2任務(wù)微調(diào) 原創(chuàng)
??從零實現(xiàn)大模型-BERT預(yù)訓(xùn)練??
??從零實現(xiàn)大模型-BERT微調(diào)??
我們在BERT微調(diào)那篇文章中提到,許多NLP任務(wù)(如情感分析和問答)都依賴于上下文理解能力。而像BERT這種雙向模型具有較強(qiáng)的上下文理解能力,因此非常適合用于任務(wù)微調(diào),即針對某個具體任務(wù)進(jìn)行微調(diào)。
??從零實現(xiàn)大模型-GPT2預(yù)訓(xùn)練??
??從零實現(xiàn)大模型-GPT2指令微調(diào)??
而像GPT這種自回歸模型,在預(yù)訓(xùn)練完成后會進(jìn)行一個指令微調(diào)過程,用于跟隨人類指令,然后通過指令去完成不同的任務(wù)(翻譯,總結(jié))。
那GPT能否像BERT那樣,直接微調(diào)用于完成某個具體任務(wù)呢?雖然BERT更適合,但GPT確實也可以。
今天我們就基于之前的GPT2預(yù)訓(xùn)練模型,使用一個垃圾郵件數(shù)據(jù)集,來微調(diào)一個郵件分類模型。
1.垃圾郵件識別
傳統(tǒng)ML方法
當(dāng)然,也可以通過傳統(tǒng)的機(jī)器學(xué)習(xí)方法實現(xiàn)垃圾郵件分類,例如,貝葉斯分類,或者基于統(tǒng)計學(xué),事先設(shè)定一些敏感詞匯,如果郵件中出現(xiàn)了這些敏感詞匯,就認(rèn)為是垃圾郵件。
Embedding
但傳統(tǒng)機(jī)器學(xué)習(xí)方法依賴人類經(jīng)驗,既然我們正在寫大模型的文章,就得入鄉(xiāng)隨俗。
我們通過Embedding實現(xiàn)文本分類,具體來說就是計算郵件內(nèi)容的Embedding,然后計算“spam”和“none spam”的Embedding,最后通過計算余弦相似度來判斷郵件類型。
指令微調(diào)模型
其實,還有比計算Embedding更簡單的方法,如果大模型已經(jīng)經(jīng)過預(yù)訓(xùn)練、指令微調(diào)以及RLHF過程,那么就可以直接利用這種指令跟隨能力來實現(xiàn)垃圾郵件分類。
例如,我們構(gòu)造下面的prompt輸入給chatGPT。
"Is the following text 'spam'? Answer with 'yes' or 'no':"
" 'You are a winner you have been specially"
" selected to receive $1000 cash or a $2000 award.'"
以下是GPT4-o給出的答案,不僅準(zhǔn)確識別出了垃圾郵件,還遵循了人類指令輸出了“yes”。
如果只使用預(yù)訓(xùn)練模型,沒有經(jīng)過指令微調(diào),前面我們也測試過,模型雖然有輸出,但輸出只是簡單的拷貝輸入。
輸入:
Is the following text 'spam'? Answer with 'yes' or 'no': 'You are a winner you have been specially selected to receive $1000 cash or a $2000 award.'
輸出:
The following text 'spam'? Answer with 'yes' or 'no': 'You are a winner
2.任務(wù)微調(diào)
本文完整代碼如下,建議結(jié)合代碼閱讀文本。
https://github.com/AIDajiangtang/LLM-from-scratch/blob/main/GPT2_fine-tune_spam_classifier_from_scratch.ipynb
在通過Embedding實現(xiàn)文本分類時,Embedding是通過調(diào)用openAI的API得到的,其實,這個Embedding也可以看作是GPT模型輸出隱狀態(tài)的一部分。
除了用Embedding計算余弦相似度來實現(xiàn)文分類外,還可以基于隱狀態(tài)實現(xiàn)一個分類模型,也就是在模型的輸出端加一個分類頭。
準(zhǔn)備數(shù)據(jù)
訓(xùn)練數(shù)據(jù)來自公開的垃圾郵件數(shù)據(jù)集,包括文本和標(biāo)簽兩列,標(biāo)簽列中spam代表是垃圾郵件。
因為數(shù)據(jù)集中垃圾郵件數(shù)量少于正常郵件,所以要平衡正負(fù)樣本數(shù)量。
# Examine class distributions
print(df["Label"].value_counts())
Label
ham 4825
spam 747
Name: count, dtype: int64
def create_balanced_dataset(df):
num_spam = df[df["Label"] == "spam"].shape[0]
ham_subset = df[df["Label"] == "ham"].sample(num_spam, random_state=123)
balanced_df = pd.concat([ham_subset, df[df["Label"] == "spam"]])
return balanced_df
balanced_df = create_balanced_dataset(df)
print(balanced_df["Label"].value_counts())
Label
ham 747
spam 747
Name: count, dtype: int64
接下來劃分訓(xùn)練集,驗證機(jī)和測試集。
接下來是tokenization,padding或者截斷到最大長度。
最后構(gòu)造Dataloader。
加載預(yù)訓(xùn)練模型
加載gpt2預(yù)訓(xùn)練模型。
CHOOSE_MODEL = "gpt2-small (124M)"
INPUT_PROMPT = "Every effort moves"
BASE_CONFIG = {
"vocab_size": 50257, # Vocabulary size
"context_length": 1024, # Context length
"drop_rate": 0.0, # Dropout rate
"qkv_bias": True # Query-key-value bias
}
model_configs = {
"gpt2-small (124M)": {"emb_dim": 768, "n_layers": 12, "n_heads": 12},
"gpt2-medium (355M)": {"emb_dim": 1024, "n_layers": 24, "n_heads": 16},
"gpt2-large (774M)": {"emb_dim": 1280, "n_layers": 36, "n_heads": 20},
"gpt2-xl (1558M)": {"emb_dim": 1600, "n_layers": 48, "n_heads": 25},
}
BASE_CONFIG.update(model_configs[CHOOSE_MODEL])
assert train_dataset.max_length <= BASE_CONFIG["context_length"], (
f"Dataset length {train_dataset.max_length} exceeds model's context "
f"length {BASE_CONFIG['context_length']}. Reinitialize data sets with "
f"`max_length={BASE_CONFIG['context_length']}`"
)
from gpt_download import download_and_load_gpt2
model_size = CHOOSE_MODEL.split(" ")[-1].lstrip("(").rstrip(")")
settings, params = download_and_load_gpt2(model_size=model_size, models_dir="gpt2")
model = GPTModel(BASE_CONFIG)
load_weights_into_gpt(model, params)
model.eval()
再微調(diào)過程中,可以凍結(jié)大部分預(yù)訓(xùn)練模型參數(shù)。
# Freeze all model layers first
for param in model.parameters():
param.requires_grad = False
添加分類頭
在預(yù)訓(xùn)練模型的輸出端加一個二分類分類頭。
# Add a classification head
torch.manual_seed(123)
num_classes = 2
model.out_head = torch.nn.Linear(
in_features=BASE_CONFIG["emb_dim"], # 768
out_features=num_classes, # 2 (spam or not spam)
)
構(gòu)造損失函數(shù)
因為是二分類,所以構(gòu)造一個交叉熵?fù)p失函數(shù)。
def calculate_loss_batch(input_batch, target_batch, model, device):
input_batch, target_batch = input_batch.to(device), target_batch.to(device)
logits = model(input_batch)[:, -1, :] # Grab logits of last output token only!
loss = torch.nn.functional.cross_entropy(logits, target_batch)
return loss
然后開始訓(xùn)練,訓(xùn)練過程與指令微調(diào)過程基本一致。
import time
start_time = time.time()
torch.manual_seed(123)
# Create optimizer
optimizer = torch.optim.AdamW(model.parameters(), lr=5e-5, weight_decay=0.1)
# Set training epochs
num_epochs = 5
# Train the model
train_losses, val_losses, train_accs, val_accs, examples_seen = train_classifier(
model=model,
train_loader=train_loader,
val_loader=val_loader,
optimizer=optimizer,
device=device,
num_epochs=num_epochs,
eval_freq=50,
eval_iter=5,
tokenizer=tokenizer,
)
end_time = time.time()
execution_time_minutes = (end_time - start_time) / 60
print(f"Training completed in {execution_time_minutes:.2f} minutes.")
本文轉(zhuǎn)載自公眾號人工智能大講堂
原文鏈接:??https://mp.weixin.qq.com/s/n1h9JeCxV3Kq_yj0-_RS5A??
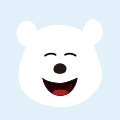